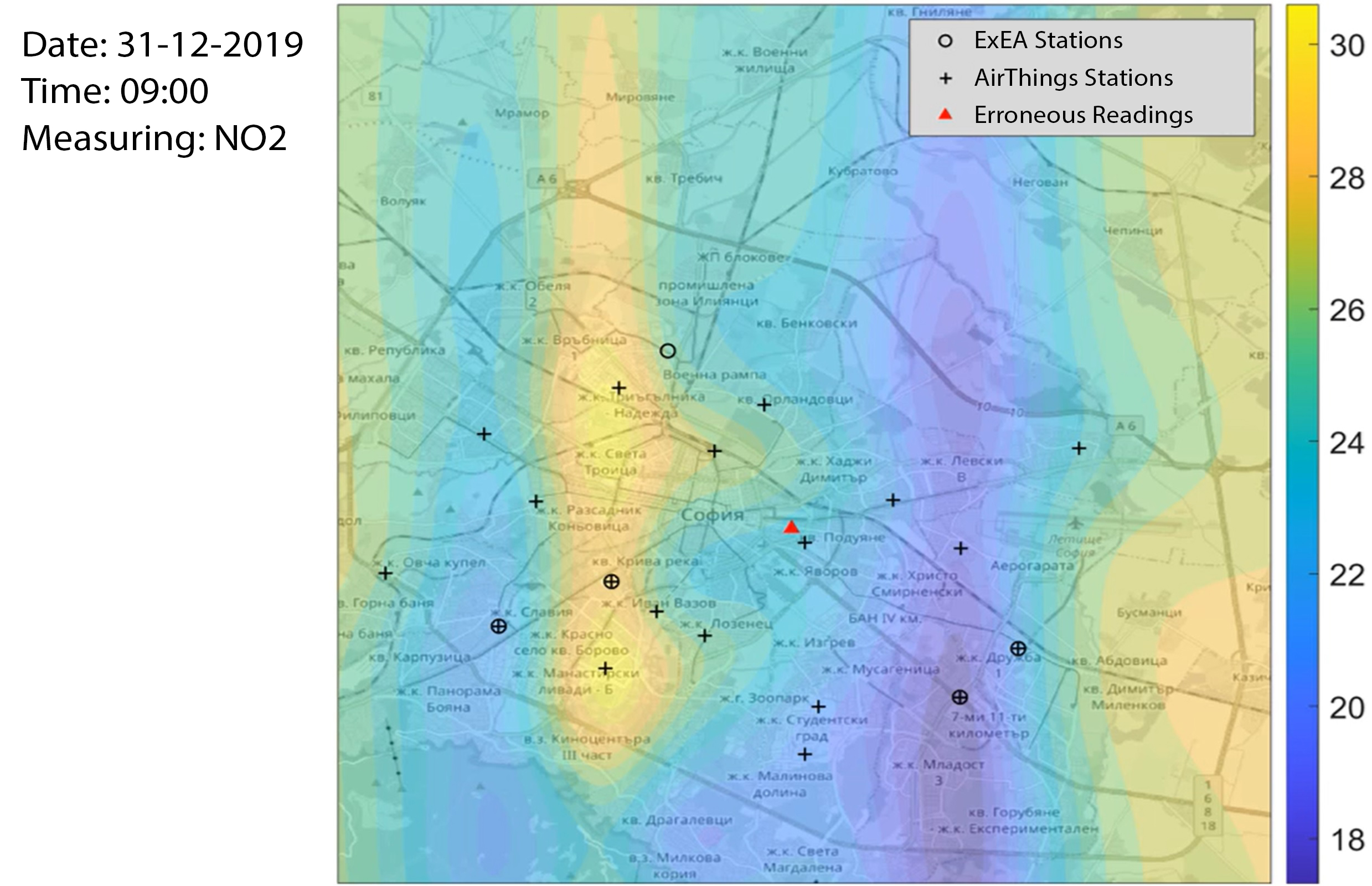
Geospatial Air Quality Prediction
Air quality monitoring is an important aspect of urban governance. High concentration levels of different gases and particulate matter can have an adverse effect on human health and standard of living in urban regions. To provide real time monitoring and analysis of long-term trends of the concentration of atmospheric pollutants, networks of sensor stations have been developed under different municipal and private initiatives. These stations measure many atmospheric properties and provide summaries for the quality of ambient air. Such measurements are made at a finite number of locations, providing pointwise data, and leaving out much of the urban area. Furthermore, different station providers use sensor equipment of varying quality, the measurements of which cannot and should not be considered directly compatible.
The aim of the geospatial air quality work is to provide a way of combining data from different sensor stations and to develop a platform for the real-time prediction of air quality throughout Sofia city. To achieve this, methods from the field of uncertainty quantification and statistical learning are used, which enable the analysis of existing sensor data in a probabilistic way.
The developed platform is envisioned to serve several purposes, including the monitoring and prediction of data-based air quality metrics, the determination of optimal placement of future sensor stations and the virtual surrogate for sensors during downtime. The platform also streams data to the computational air quality models developed at GATE to support the construction of the City Digital Twin.